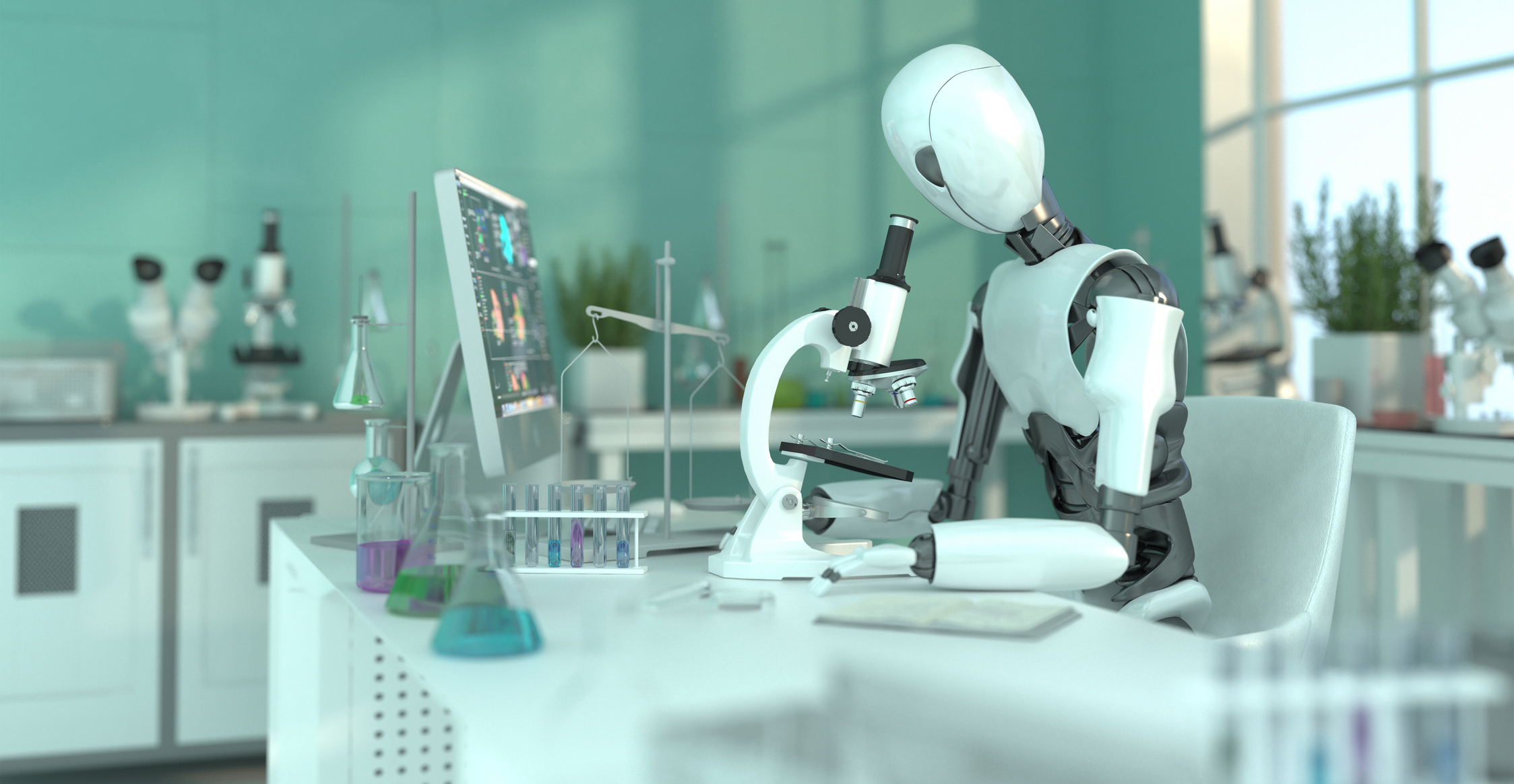
Clinical trials remain the only way to ensure the safety and efficacy of medical interventions. Yet many medical interventions fail to reach from the regulatory authority.
An analysis of clinical trial data from January 2000 to April 2019 estimated that only around 12% of drug-development trials were completed and resulted in a medical intervention being approved.
Although this trend is changing, the percentage of interventions that navigate all three stages of clinical testing and remains around 30%.
“There are many different ways that a clinical trial can go wrong,” said Professor Wray Buntine, a data scientist at Monash University in Melbourne.
Now, researchers and pharma companies are turning to data mining and AI to reduce clinical trial cycle times, save billions of dollars and expand access to experimental treatments.
Patient recruitment is the first bottleneck of clinical trials. It’s a time-consuming and expensive process.
“A lot of trials don’t recruit enough people just because [patients] don’t know they exist,” said Dr Sarvnaz Karimi, a senior research scientist at CSIRO. “But if a system could automatically match medical notes of a patient to available trials, it would save a lot of labour,” she said.
Karimi is developing a search technology to match cancer patients with oncology clinical trials using natural language processing (NLP) and information retrieval.
NLP is a branch of AI that enables computers to analyse text. Applied to oncologists’ notes, NLP could allow algorithms to search for people who would be eligible to participate in a given clinical trial.
This system’s novelty is a ranking function that uses information retrieval techniques designed to surface the most relevant information within large datasets. The function ranks clinical trials that best match the patient to the top of the list, “just like Google search gives you your top-ranking results when you type in a query,” said Karimi.
But harvesting information automatically is especially complicated for medical texts, said Professor Jon Patrick, CEO at Health Language Analytics.
Information needs to be gathered and linked across various types of documents, such as radiology, pathology or mammography tests, discharge summaries and billing records.
Medical documents are often unstructured, don’t follow grammatical rules, miss valuable information and contain abbreviations and acronyms. “Translating them into a form that makes them computable, and matching them against any NLP engine, is quite difficult,” Patrick said. “So [the AI] has got to get things right at multiple levels to give high-level accuracy,” he added.
Commercial search engines, such as Google and Amazon, have an accuracy range of 70 to 80%, said Patrick. “[But] in the clinical setting, it has to be much more accurate than the technology people are most familiar with,” he said.
“It’s never like a human, it’s never perfect,” added Karimi. But latest advances are getting us closer to understanding medical language at a very high level of accuracy, she said.
Another area where AI could improve clinical trials is the study design.
“The thing about biology and health is that they’re incredibly complex,” said Buntine. He said that factors relevant to our health, such as nutrition, the environment we live in, the job we do, and our physical activity levels, are rarely considered in trials.
A binary design – drug versus placebo, for example – isn’t enough, said Buntine.
“Due to the complexities of the modern world, drugs, drug interactions, our models of human biology and all the environmental factors that we cannot control, it’s now essential to introduce data mining to come up with reasonable options,” he said.
The rapidly increasing amounts of medical data, including those provided by electronic medical records, wearable devices and environmental sensors, means that scientists have a growing pool of data they can mine to train sophisticated machine learning algorithms that support multidimensional study designs.
But this gigantic collection of information raises questions around privacy.
“[Privacy] can be dealt in a number of ways,” said Patrick.
Often the developers don’t need to access the data. Instead, their AI system is integrated within the client’s database – for example, a hospital’s records – so that patients’ information never leaves the clinic.
Another strategy is to anonymise records so that no one would be able to track patients back.
Many privacy technologies such as cryptography, blockchains and federated learning have been developed to keep people’s data safe while allowing researchers to use them in their AI systems.
“There are fabulous technologies for trying to enforce privacy,” said Buntine, but ultimately it is up to those who use the data to do the right thing.
“There are views that data collected by hospitals and other health organisations should be made available to the research community so that we can speed up the research. And then the opposing view is that we are enabling big tech and big pharma companies to get more and more control over our lives. I don’t think there’s an answer to that sort of dilemma,” said Patrick. “You’ve got to decide which you think is more important.”